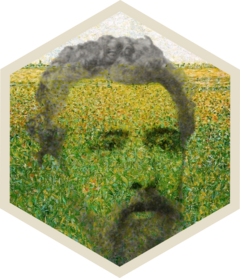
Run ComBat on Seurat's Assay5 object through IntegrateLayers
Source: R/Combat.R
CombatIntegration.Rd
A wrapper to run ComBat
or
ComBat_seq
on multi-layered Seurat V5 object
Usage
CombatIntegration(
object,
orig = NULL,
groups = NULL,
groups.name = NULL,
layers = "data",
scale.layer = "scale.data",
features = NULL,
reconstructed.assay = "combat.reconstructed",
key.assay = "combat_",
combat.function = c("combat", "combat_seq"),
use.scaled = FALSE,
verbose = TRUE,
...
)
Arguments
- object
A
Seurat
object (or anAssay5
object if not called byIntegrateLayers
)- orig
DimReduc
object. Not to be set directly when called withIntegrateLayers
, useorig.reduction
argument instead- groups
A named data frame with grouping information. Preferably one-column when
groups.name = NULL
- groups.name
Column name from
groups
data frame that stores grouping information. Ifgroups.name = NULL
, the first column is used- layers
Name of the layers to use in the integration
- scale.layer
Name of the scaled layer in
Assay
- features
Vector of feature names to input to the integration method. When
features = NULL
(default), theVariableFeatures
are used. To pass all features, use the output ofFeatures()
- reconstructed.assay
Name for the
assay
containing the corrected expression matrix- key.assay
Optional key for the new combat assay. Format: "[:alnum:]*_"
- combat.function
ComBat implementation to use. One of combat, combat_seq. Note that ComBat_seq is an improved model from ComBat but requires a dense matrix. Sparse to dense matrix conversion can be memory-intensive.
- use.scaled
By default the layer passed to the
layer
argument is used. Whenuse.scaled = TRUE
, thescale.layer
is input to ComBat.- verbose
Print messages. Set to
FALSE
to disable- ...
Additional arguments passed on to ComBat or ComBat_seq.
Value
The function itself returns a list containing:
a new Assay of name
reconstructed.assay
(key set toassay.key
) with corrected cell counts.
When called via IntegrateLayers
, a Seurat object with
the new assay is returned
Note
This function requires the sva (Surrogate Variable Analysis) package to be installed
References
Johnson, W. E., Li, C. & Rabinovic, A. Adjusting batch effects in microarray expression data using empirical Bayes methods. Biostatistics 8, 118–127 (2006). DOI
Zhang, Y., Parmigiani, G. & Johnson, W. E. ComBat-seq: batch effect adjustment for RNA-seq count data. NAR Genomics and Bioinformatics 2 (2020). DOI
Examples
if (FALSE) { # \dontrun{
# Preprocessing
obj <- UpdateSeuratObject(SeuratData::LoadData("pbmcsca"))
obj[["RNA"]] <- split(obj[["RNA"]], f = obj$Method)
obj <- NormalizeData(obj)
obj <- FindVariableFeatures(obj)
obj <- ScaleData(obj)
obj <- RunPCA(obj)
# After preprocessing, we integrate layers based on the "Method" variable:
obj <- IntegrateLayers(object = obj, method = CombatIntegration,
verbose = TRUE, layers = "data", scale.layer = NULL,
features = VariableFeatures(
FindVariableFeatures(obj, nfeatures = 5e3)
))
# We can also change parameters such as the input data.
# Here we use the scale data, the ComBat implementation and we use the cell
# labels as a "biological condition of interest" (/!\ long):
obj <- IntegrateLayers(object = obj, method = CombatIntegration,
verbose = TRUE, features = VariableFeatures(obj),
use.scaled = FALSE, combat.function = 'combat_seq',
group = obj[[]]$CellType, groups = obj[[]],
groups.name = "Method", layers = "counts")
} # }